The role of data in AI solutions
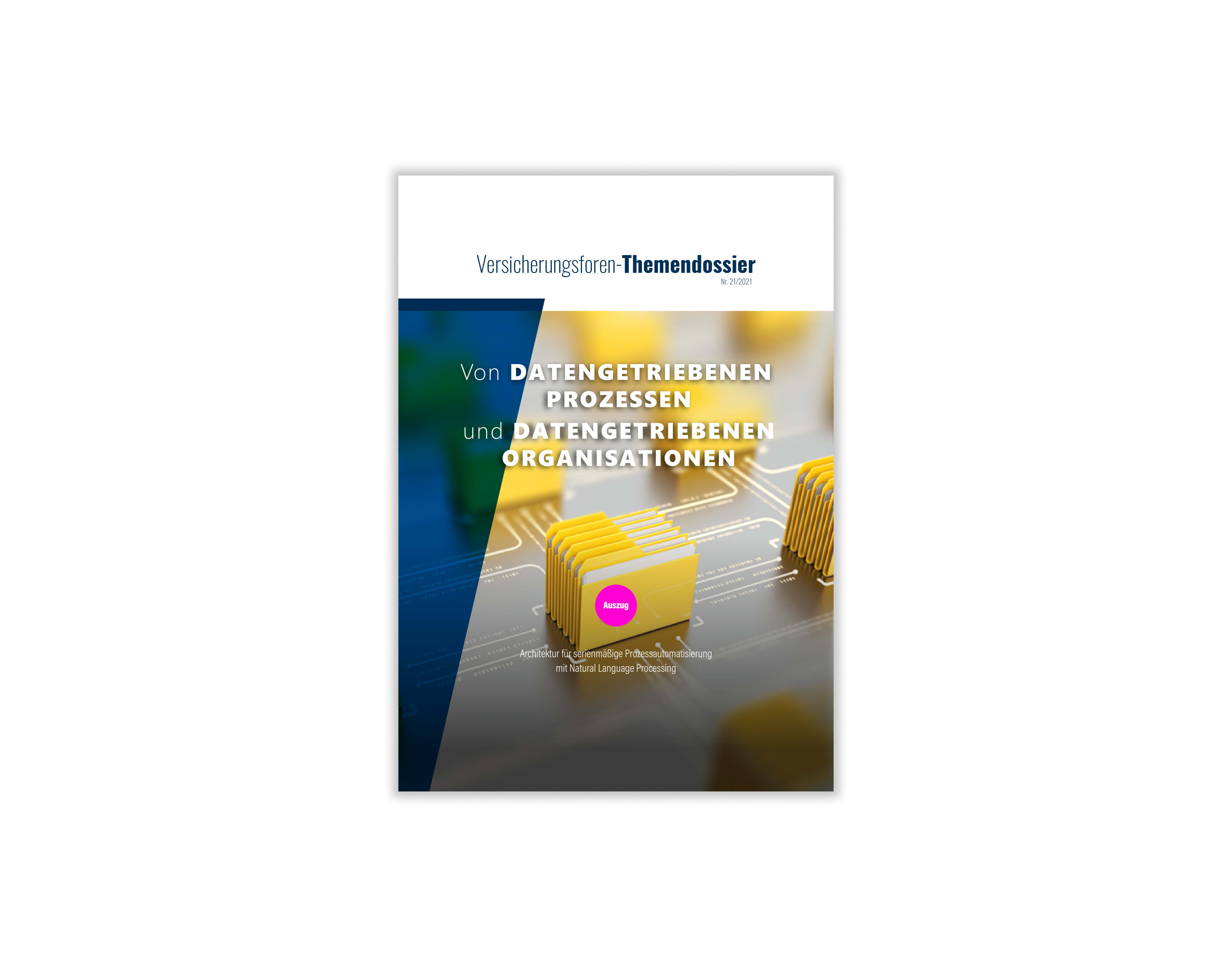
This is an excerpt from the guest article “How CTOs Can Convince the Other C-Level of AI” in the Versicherungsforen Leipzig dossier. Click here to download the full article (in German) as a PDF.
–
Every AI solution needs a basic set of training data. But there are differences depending on the application. Classic machine learning models need sufficient historical data. Without this, high-performance processing is not feasible.
The situation is different in the area of reinforcement learning (RL), where the algorithm explores its environment without any training and learns “the game” on its own. For example, Bandit algorithms from the RL domain are used to design systems to automatically adapt to changing environmental conditions.
It’ s a different story again in Natural Language Processing, where, for example, with the appropriate technology and expertise, it is possible to generate training data synthetically. In this way, use cases with >200 sample documents can be realized on a regular basis.
This regularly results in new use cases where a lot of information is available in text form, but cannot be used in a structured way as data by the existing systems. Knowledge that is de facto lost to the company operationally. Examples include capturing application data as it is received, detecting silent cyber in commercial contracts, or evaluating claims reports.
This is where NLP solutions come into play. Trained with a few sample documents and redundantly secured by a fallback process to the clerk (human-in-the-loop), these algorithms can semantically evaluate texts.
**In short, data is the foundation of any modern IT solution. Where once the focus was on mapping processes, today it is often a matter of outsourcing entire heuristics to algorithms. The good news is that the ways in which data can be obtained and processed are becoming increasingly simple.